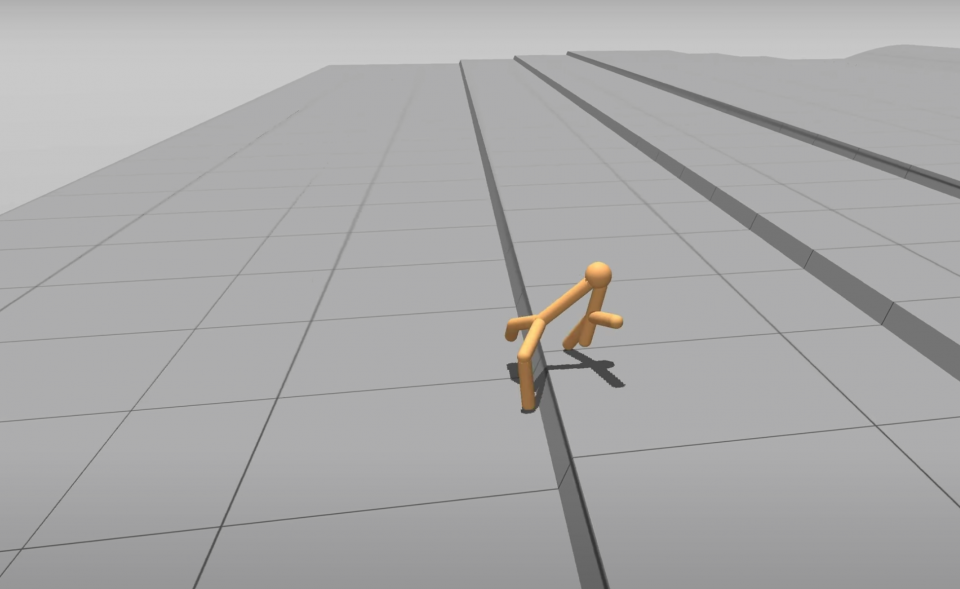
An in silico playground for creatures that learn, mutate, and evolve establishes the importance of morphological intelligence and suggests a new way to optimize embodied AIs.
Katharine Miller
Animals have embodied smarts: They perform tasks that their bodies are well designed for. That’s because the intelligence of every animal species evolved in tandem with its physical form as it interacted with its environment. Thus, spiders weave webs with their spindly legs, beavers slap their broad tails to sound an alarm, cheetahs run fast to catch zebras, and humans have opposable thumbs for grasping tools.
Artificial intelligence is quite smart as well. But unlike animal smarts, AI is often disembodied. Natural language processing and other types of machine learning, for example, are typically done on silicon chips inside computers, with no physical manifestation in the world. And while computer vision requires cameras or sensors, it usually does so independently of any physical form.
A team of researchers at Stanford wondered: Does embodiment matter for the evolution of intelligence? And if so, how might computer scientists make use of embodiment to create smarter AIs?
To answer these questions, they created a computer-simulated playground where arthropod-like agents dubbed “unimals” (short for universal animals and pronounced “yoo-nimals”) learn and are subjected to mutations and natural selection. The researchers then studied how having virtual bodies affected the evolution of the unimals’ intelligence.
Read the full study, "Embodied Intelligence Via Learning and Evolution"
“We're often so focused on intelligence being a function of the human brain and of neurons specifically,” says Fei-Fei Li, a member of the research team and co-director of the Stanford Institute for Human-Centered AI (HAI). “Viewing intelligence as something that is physically embodied is a different paradigm.”
Their findings, detailed in the journal Nature Communications, suggest embodiment is key to the evolution of intelligence: The virtual creatures’ body shapes affected their ability to learn new tasks, and morphologies that learn and evolve in more challenging environments, or while performing more complex tasks, learn faster and better than those that learn and evolve in simpler surroundings. In the study, unimals with the most successful morphologies also picked up tasks faster than previous generations — even though they began their existence with the same level of baseline intelligence as those that came before.
“To our knowledge, this is the first simulation to show that what you learn within a lifetime can be sped up just by changing your morphology,” says study co-author Surya Ganguli, an associate professor of applied physics in the School of Humanities and Sciences and an associate director at HAI.
The team’s algorithm, called DERL (Deep Evolutionary Reinforcement Learning), could help researchers design robots that are optimized to perform real-world tasks in real-world environments. “If we want these agents to make our lives better, we need them to interact in the world we live in,” said study first author and computer science graduate student Agrim Gupta.
The Unimal Universe
To understand the evolution of embodied intelligence, the team varied not only the unimals’ body shapes but also their training environments and the tasks they performed. And all of these variables were much more complex than in prior work, Ganguli says. “This allowed us to look at a lot more scientific questions than had been done previously.”
The team also adopted a tournament-style Darwinian evolutionary scheme to ensure that each unimal morphology had more than one opportunity to succeed and be passed on to the next generation. This approach served to maintain diversity in the unimal population and also reduced the computational cost of the simulations.
Each simulation began with 576 unique unimals consisting of a “sphere” (the head) and a “body” composed of different numbers of jointed, cylindrical limbs arranged in various arrangements. Each unimal sensed the world in the same way and started out with the same neural architectures and learning algorithms. In other words, all of the unimals began their virtual lives with the same level of smarts — only their body shapes differed.
Each unimal then went through a learning phase in which it either navigated flat terrain or a more challenging terrain that included blocky ridges, stairsteps, and smooth hills. Some of the unimals had to move a block to a target location across the variable terrain. After training, each unimal entered a tournament with three other unimals that were trained on the same environment/task combo. The winner was selected to produce a single offspring that underwent a single mutation involving changes to limbs or joints before facing the same tasks as its parents. All of the unimals (including the winners) participated in multiple tournaments, aging out only as new offspring emerged.
After 4,000 different morphologies had been trained, the researchers ended the simulation. At that point, the surviving unimals had gone through, on average, 10 generations of evolution, and the successful morphologies were surprisingly diverse, including bipeds, tripeds, and quadrupeds with and without arms.
The Gladiator Challenge
After completing three evolutionary runs per environment (4,000 morphologies per run), Gupta and his colleagues selected the top 10 performing unimals from each environment and trained them from scratch on eight brand-new tasks such as navigating around obstacles, manipulating a ball, or pushing a box up an incline.
The upshot: Gladiators that had evolved in variable terrain performed better than those that evolved in flat terrain, and those that had evolved to manipulate a box in variable terrain performed the best. The most successful unimals also learned faster both individually (by achieving better performance with less training) and across generations. Indeed, after 10 generations, the most successful unimal morphologies became so well adapted that they learned the same task in half as much time as their earliest ancestor.
This is consistent with a hypothesis made in the late 1800s by American psychologist James Mark Baldwin, who conjectured that the ability to learn things that have an adaptive advantage could in fact be passed down through Darwinian natural selection. In essence, Gupta says, “Nature selects for body changes that make acquisition of advantageous behavior faster.”
Making Smarter AIs
Since agents that evolve in more complex environments learned new tasks faster and better, Gupta and his colleagues believe that allowing simulated embodied agents to evolve in increasingly complex environments will provide insights for developing robots that can learn to perform multiple tasks in the real world.
Humans don't necessarily know how to design robot bodies for strange tasks such as crawling through a nuclear reactor to extract waste, providing disaster relief after an earthquake, guiding a nanorobot through the human body, or even doing domestic tasks such as washing dishes or folding laundry.
“Perhaps the only way forward is to allow evolution to design these robots,” Ganguli says.
Add comment
Comments