So many of Google’s technology developments have had major impacts in the commercial world but the company’s impact on scientific computing has been far less pronounced. Teams at the search engine giant are trying to bridge that gap by creating software tools that blend the best of two worlds — traditional numerical simulations and modern machine learning.
Stephan Hoyer has been working at this intersection of scientific computing and deep learning at Google since 2016, specifically trying to find ways to move ML and HPC closer together in climate and weather modeling. Before that, he worked on climate and risk models at the Climate Corporation following a Ph.D in physics at UC Berkeley. In short, he has a good sense of what works for traditional numerical simulations and where machine learning might fit.
Hoyer and team in the applied sciences group at Google are focusing on “differentiable programming” as the route to meshing the worlds of traditional HPC and ML. “Numerical methods are interpretable and generalizable and neural networks work well for approximation,” he says. “In physics, there is always some level of approximation. Our goal is how to best artful inject a bit of machine learning just where it’s needed to improve results.”
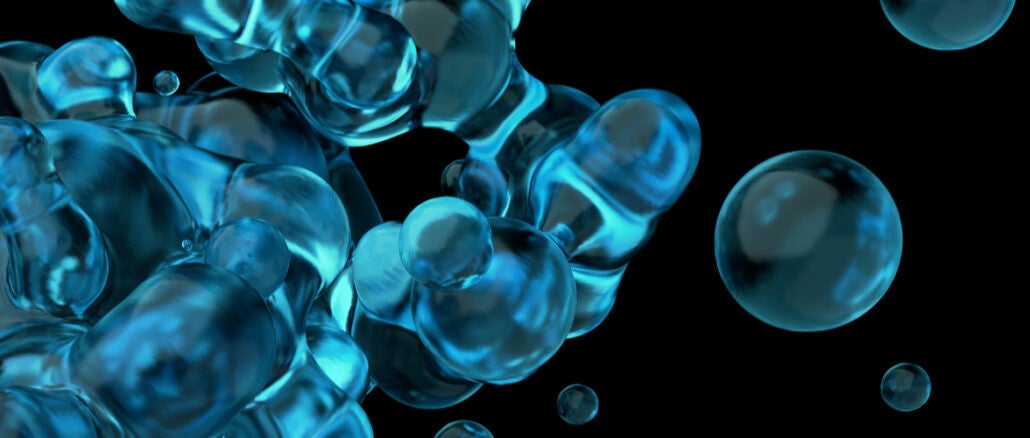
Add comment
Comments